Understanding Convolutional Neural Network Stock Market Prediction
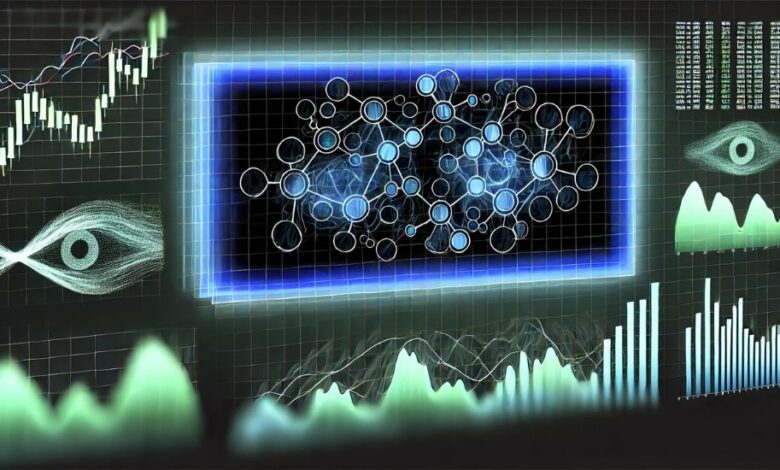
In recent years, technological advancements have revolutionized the stock market industry, especially in the realm of stock price prediction. One key area where artificial intelligence (AI) plays a transformative role is through convolutional neural networks (CNNs). A convolutional neural network stock market prediction model utilizes machine learning techniques to analyze large volumes of financial data, identify patterns, and make predictions. Here, USA Magzines dives deep into the concept of convolutional neural network stock market prediction, exploring how CNNs contribute to financial forecasting and impact trading strategies.
What is a Convolutional Neural Network?
Convolutional neural networks are a type of deep learning model initially developed for image and video processing. Inspired by the human visual cortex, CNNs can identify spatial patterns, making them particularly useful in applications that require pattern recognition. However, the versatility of CNNs extends beyond image processing, and they are increasingly being used in fields like natural language processing, medical diagnostics, and, more recently, in stock market prediction.
When applied to the stock market, CNNs help recognize complex patterns in historical price data, volume, and other financial indicators, facilitating accurate predictions and trading insights. USA Magzines explores how convolutional neural network stock market models leverage deep learning’s power for financial gains.
Why Use CNNs in the Stock Market?
Pattern Recognition in Financial Data
The primary advantage of using CNNs in the stock market lies in their exceptional ability to identify patterns. The stock market is full of complex, multi-dimensional data, ranging from time-series prices to historical trends. A convolutional neural network stock market model effectively captures these complex patterns, even in highly volatile data. This enables financial analysts and trading systems to predict trends, calculate risks, and make informed investment decisions.
Better Accuracy in Prediction
The stock market’s predictive nature relies heavily on algorithms that can account for non-linear data, and CNNs are ideal for this purpose. Unlike traditional machine learning models, which might overlook the intricate patterns in financial time-series data, CNNs use a layered approach to extract and process data, improving prediction accuracy. USA Magzines notes that financial institutions and individual investors are increasingly adopting CNNs to gain a competitive edge.
How Convolutional Neural Networks Work in Stock Market Analysis
Input Layer and Data Preprocessing
In a convolutional neural network stock market model, data preprocessing plays a vital role. Raw stock market data is often noisy and irregular, requiring normalization and transformation into suitable formats. Time-series data, for example, is segmented into windows for CNNs to analyze, with historical price data, technical indicators, and sometimes sentiment analysis inputted as features.
Convolutional Layers for Pattern Detection
Convolutional layers form the backbone of CNNs, working to identify patterns through a process of filtering. In stock market applications, these filters extract features like daily highs and lows, trading volume fluctuations, and moving averages. By applying these filters over the time-series data, CNNs can isolate meaningful patterns from historical stock prices, allowing for more refined predictions.
Pooling and Fully Connected Layers
Pooling layers help reduce the data’s complexity, ensuring the model only retains the most relevant information, enhancing computational efficiency. Following the pooling layers, fully connected layers integrate all extracted features, enabling the convolutional neural network stock market model to classify data or make predictions. By compressing information and focusing on key features, CNNs provide a robust framework for stock price prediction.
Types of CNN Architectures in Stock Market Prediction
1. 1D Convolutional Neural Networks
One-dimensional CNNs are particularly useful in stock market prediction since they process sequential time-series data efficiently. These models apply convolutional operations over a single dimension, such as time or daily price, capturing sequential dependencies that are crucial for financial forecasting.
2. Hybrid CNN-LSTM Models
Combining CNNs with Long Short-Term Memory (LSTM) networks offers another effective approach for stock market analysis. LSTMs are well-suited to handle time-series data, accounting for long-term dependencies that CNNs alone might overlook. In a convolutional neural network stock market hybrid model, CNN layers capture short-term patterns, while LSTM layers consider long-term trends, delivering a more comprehensive prediction model.
3. Multi-Channel CNNs
Multi-channel CNNs allow for the simultaneous analysis of various financial indicators, such as stock prices, volume, sentiment analysis, and macroeconomic factors. By feeding each data type through separate CNN channels, this architecture captures diverse patterns and relationships, making it particularly advantageous for complex stock market forecasting models.
Advantages of CNNs in Stock Market Prediction
Automation of Complex Tasks
One of the biggest advantages of convolutional neural network stock market prediction models is their ability to automate complex analytical tasks. Manual stock analysis requires extensive knowledge, skill, and time. CNNs automate this process, enabling rapid decision-making and allowing analysts to focus on strategy rather than data interpretation.
Improved Decision-Making for Investors
Using CNNs enhances decision-making accuracy, as they can process vast datasets and reveal patterns hidden from human observation. With a well-trained convolutional neural network stock market model, investors can make more accurate predictions about stock movements, ultimately improving profitability and reducing risk.
Ability to Handle Non-linear Data
Traditional linear models often fail to capture the stock market’s non-linear characteristics. CNNs are inherently capable of modeling non-linear relationships, adapting to changes in the market and predicting future trends with higher accuracy.
Challenges of Using CNNs in Stock Market Prediction
High Computational Demand
Convolutional neural network stock market models require substantial computational resources, making them expensive to implement and maintain. Training a CNN demands high-performance hardware, particularly when dealing with large datasets and complex architectures like CNN-LSTM hybrids.
Risk of Overfitting
Overfitting is a common issue in CNN models, where the model performs well on training data but fails to generalize to new data. In stock market prediction, this risk is particularly high due to market volatility. USA Magzines advises careful regularization and cross-validation when developing a convolutional neural network stock market model to avoid overfitting and improve real-world performance.
Limited Interpretability
While CNNs are powerful, they operate as “black boxes,” making it difficult to understand how they make specific predictions. Investors might be hesitant to rely on a convolutional neural network stock market model without a clear explanation of its decision-making process.
Real-World Applications of CNNs in the Stock Market
High-Frequency Trading (HFT)
High-frequency trading firms leverage CNNs to analyze vast amounts of financial data in real time, making split-second trading decisions. The convolutional neural network stock market models used in HFT extract patterns in price fluctuations, allowing for high-speed decision-making and increased profitability.
Algorithmic Trading
Algorithmic trading strategies also benefit from CNN-based predictions. These models can execute trades based on predefined criteria, such as moving averages or RSI levels, which CNNs analyze to forecast stock trends. USA Magzines highlights CNNs’ impact on algorithmic trading by providing a data-driven basis for trading strategies.
Risk Management and Financial Planning
Banks and financial institutions utilize CNNs to assess risks and make better financial planning decisions. By forecasting potential market downturns or upswings, convolutional neural network stock market models contribute to effective risk management strategies, helping institutions safeguard assets.
Conclusion
Convolutional neural network stock market prediction models have transformed the financial industry by introducing a powerful tool for forecasting and decision-making. From high-frequency trading to risk management, CNNs offer a way to process and interpret the complex patterns in stock data, giving investors an advantage in a highly competitive field. USA Magzines believes that as technology advances, CNN applications in the stock market will continue to expand, delivering more sophisticated and accurate forecasting tools.
As the stock market becomes increasingly complex, the role of AI-driven models like CNNs will only grow, driving the future of financial technology and shaping the landscape of modern trading.